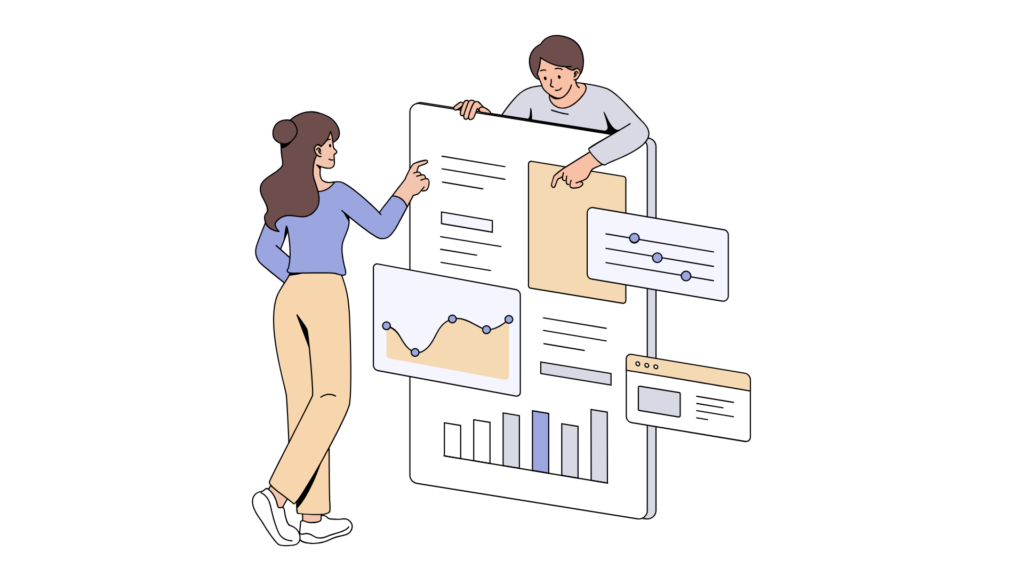
Turning raw data into actionable insights is essential for making informed business decisions and driving strategic growth. Big data consists of vast amounts of information collected from multiple sources, such as customer interactions, Internet of Things (IoT) devices, and digital platforms. Without a structured approach, this data remains overwhelming and underutilized.
This guide explores effective strategies for analyzing big data, implementing data-driven decision-making, and leveraging analytics tools to unlock business potential.
Understanding Big Data and Its Challenges
Big data analytics has transformed how businesses operate, offering deeper insights into customer behavior, operational efficiency, and market trends. However, handling vast amounts of data comes with its own set of challenges. To fully leverage its potential, businesses must first understand what big data entails and the obstacles associated with its management.
Big data is characterized by three fundamental aspects, commonly known as the Three Vs:
- Volume: The sheer amount of data generated every second from digital interactions, transactions, and connected devices.
- Velocity: The speed at which data is created and processed.
- Variety: Data comes in multiple formats, including structured (databases, sales records) and unstructured (social media posts, customer reviews, emails).
Despite the availability of vast amounts of data, 85% of business data is never used for decision-making. Many companies collect data without clear strategies for analysis, leading to wasted potential. Dirty data costs businesses $3.1 trillion annually in wasted resources (IBM), emphasizing the need for data validation and cleansing processes.
Forbes reports that 74% of companies lack a cohesive data strategy, resulting in siloed information and inefficiencies. A well-defined approach to data management is crucial for maximizing big data’s value.
Steps to Convert Big Data into Actionable Insights
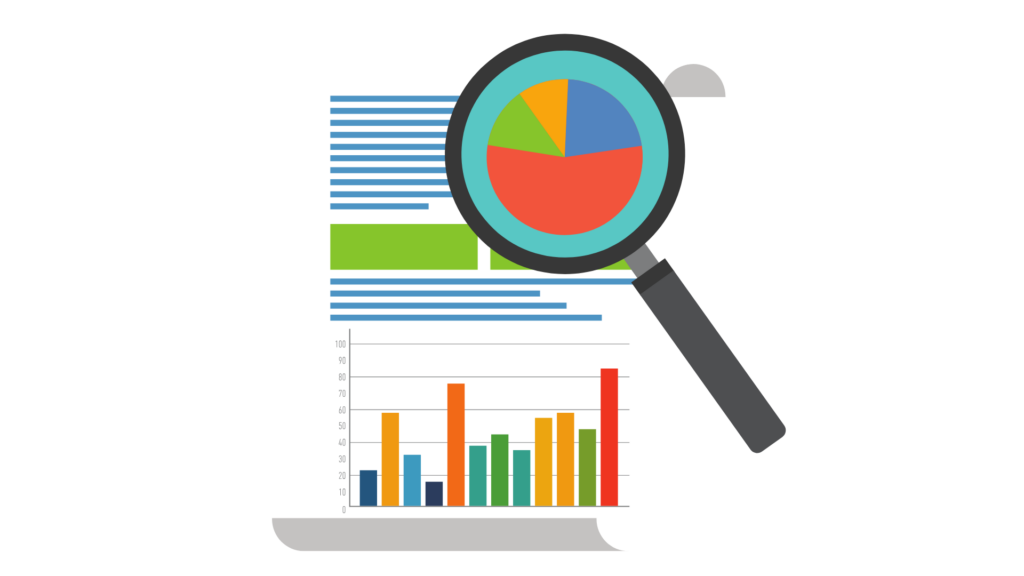
Transforming big data into meaningful business decisions requires a structured approach. The following steps outline how companies can efficiently convert raw data into insights that drive growth and innovation.
1. Define Business Goals & Data Strategy
Before diving into data analysis, businesses must establish clear objectives. Without a defined strategy, data analysis can become aimless, leading to irrelevant insights. A strong data strategy aligns analytics efforts with business priorities.
Examples of data-driven business objectives:
- Improve Customer Retention
- Optimize Supply Chain Operations
- Increase Marketing ROI
Netflix is a prime example of a company effectively leveraging big data. By analyzing viewing habits and preferences, Netflix’s recommendation engine increases viewer retention by 80% through personalized content (Wired).
2. Collect and Centralize Data from Multiple Sources
Big data originates from various sources, and consolidating it is crucial for generating accurate insights. Businesses must integrate information from different channels to get a holistic view of customer behavior and operational performance. Key sources of big data:
- CRM Platforms: Salesforce, HubSpot (customer interactions and sales data).
- Social Media Analytics: Facebook Insights, Twitter Analytics (engagement metrics and audience insights).
- Web Analytics: Google Analytics (user behavior, traffic patterns).
- Transactional Data: E-commerce platforms like Shopify, and Magento (purchase history and revenue trends).
3. Clean and Standardize Data for Accuracy
Raw data is often incomplete, inconsistent, or duplicated. Without proper data cleansing, insights derived from messy data can be misleading. Cleaning and standardizing data improves accuracy and ensures reliable analytics. Data cleaning best practices:
- Remove duplicate records to prevent skewed analysis.
- Standardize formats for consistency (e.g., uniform date formats, consistent category labels).
- Identify missing values and outliers that could distort results.
Companies that invest in better data management see up to a 40% boost in productivity. High-quality data enables faster, more informed decision-making.
4. Use Advanced Analytics & AI to Identify Patterns
Once data is clean and structured, businesses can leverage advanced analytics and AI to extract actionable insights. Different types of analytics serve different purposes, from understanding past trends to predicting future outcomes. Types of big data analytics:
- Descriptive Analytics: Answers “What happened?” by analyzing historical data (e.g., revenue trends over time).
- Diagnostic Analytics: Explores “Why did it happen?” by identifying causes of trends (e.g., customer churn analysis).
- Predictive Analytics: Forecasts “What will happen next?” using AI-driven models (e.g., sales predictions based on seasonal trends).
- Prescriptive Analytics: Suggests “What should be done?” by providing actionable recommendations (e.g., AI-powered product recommendations).
Turning Insights into Actionable Business Strategies
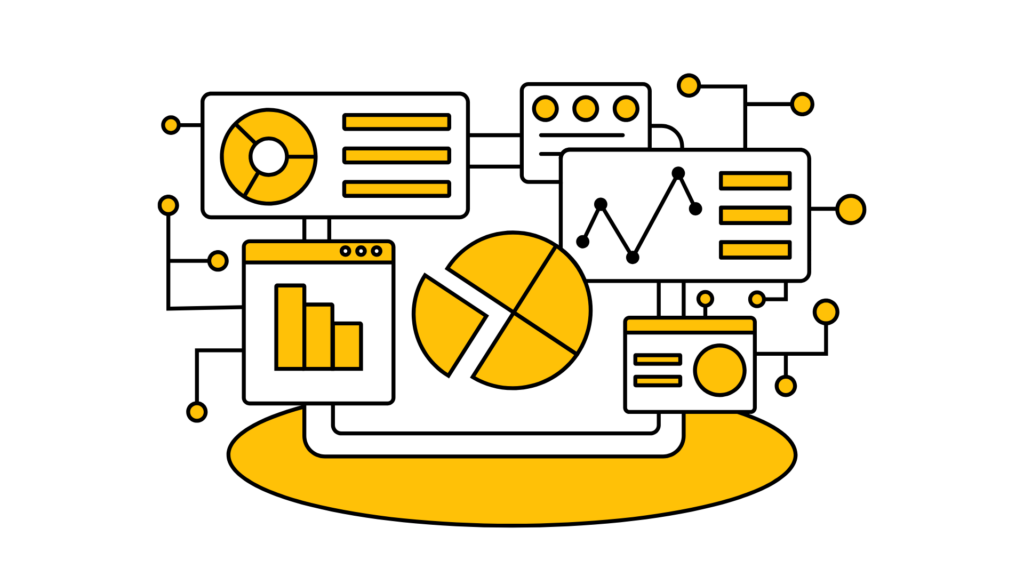
Delivering personalized experiences is key to customer retention and revenue growth. Starbucks analyzes purchase history and location data to personalize mobile app promotions, resulting in a 300% increase in app engagement. Customers receive tailored rewards and suggestions based on their past orders, enhancing brand loyalty.
Effective customer segmentation helps businesses create targeted messaging and personalized experiences for different audience groups. Segments are typically based on:
- Behavior: How users interact with a brand (e.g., frequent buyers vs. occasional shoppers).
- Demographics: Age, location, gender, income level.
- Spending Patterns: Purchase frequency, average order value, product preferences.
Personalized marketing campaigns deliver 5-8x ROI compared to broad, non-targeted campaigns. Machine learning algorithms analyze user behavior to optimize ad placements and budget allocation. Airbnb implemented machine learning to analyze user interactions, leading to a 30% increase in ad effectiveness.
Common Pitfalls & How to Avoid Them
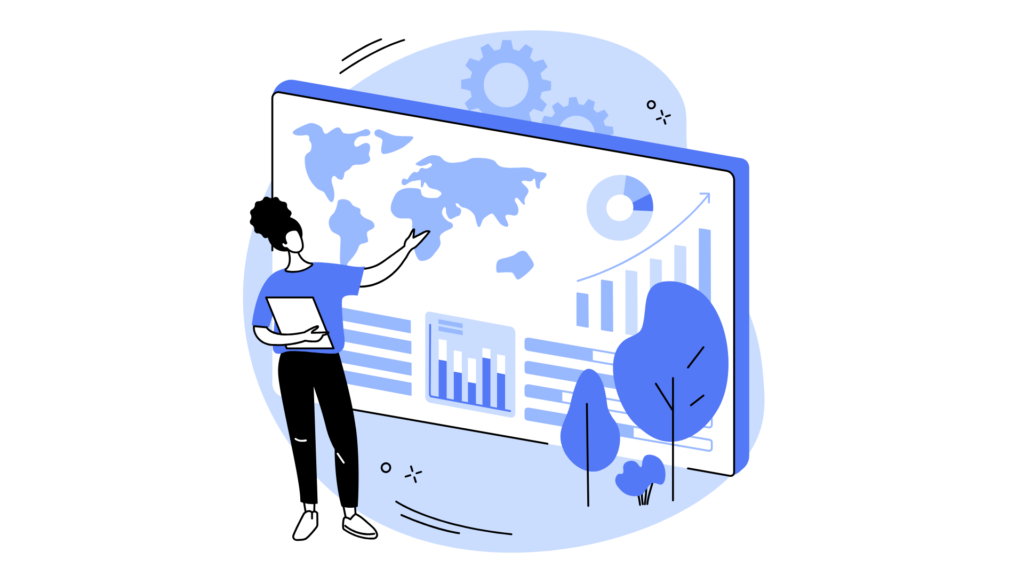
To maximize the value of data, companies must avoid these pitfalls and implement best practices for strategic data utilization.
1. Relying on Data Without Context
One of the biggest mistakes businesses make is using data in isolation without considering the broader business context. Numbers alone don’t tell the full story—qualitative insights, industry trends, and customer feedback must also be factored in.
How to avoid this pitfall:
- Combine quantitative data (numbers and statistics) with qualitative insights (customer interviews, market research).
- Always validate insights with business knowledge and human judgment before making strategic decisions.
- Ensure AI-driven predictions are regularly audited to prevent bias and unintended consequences.
2. Ignoring Data Privacy & Compliance
As businesses collect and analyze vast amounts of data, they must prioritize data security and regulatory compliance. Privacy laws like GDPR (General Data Protection Regulation) and CCPA (California Consumer Privacy Act) impose strict requirements on data handling. Companies failing to comply with these regulations face fines of up to 4% of annual revenue (EU GDPR).
3. Not Aligning Data Insights with Business Goals
Data is only valuable if it drives meaningful business outcomes. However, 60% of companies struggle to align their data strategies with business objectives. Without clear alignment, businesses risk collecting large amounts of data without extracting actionable insights.
Define Key Performance Indicators (KPIs) that align with business goals (e.g., customer acquisition cost, revenue growth, retention rates). Ensure data-driven decisions translate into measurable improvements in business performance.
Regularly review and refine analytics strategies to stay aligned with evolving company objectives. By integrating context, compliance, and strategic alignment, businesses can leverage big data effectively to drive smarter decisions and sustainable growth.
Conclusion: Driving Business Growth with Actionable Insights
Companies that effectively utilize data analytics can optimize customer experiences, streamline operations, and make smarter strategic decisions. Organizations that leverage big data strategically will outperform competitors, improve operational efficiency, and lead innovation in their industries. As data continues to shape the future of business, those who invest in the right tools, strategies, and governance frameworks will remain ahead in an increasingly competitive digital landscape.
Free Google Analytics Audits
We partner with Optimo Analytics to get free and automated Google Analytics audits to find issues or areas of improvement in you GA property.